Artificial Intelligence is changing all sorts of industries from farming to finance, and sport is no different. The AI impact on sports will be profound, particularly when it comes to sports data science analytics. Artificial Intelligence is helping teams in every aspect of their management, from recruiting talent and keeping players fit to improved training and in-game performance. For many people, AI means little more than ChatGPT and funny images but the truth is this is such a thin sliver of AI that it overlooks so many groundbreaking new technologies. Let's take a look at how some of these AI technologies are being applied to sports.
AI Performance Analytics in Sports
Sports analytics was originally very rudimentary and really only in the last 20 years has the analysis become formalized. Sports analytics was popularized by the movie Moneyball, based on the book of the same name, which detailed a groundbreaking new approach to baseball analytics. The gist of the approach was that The Oakland A’s should not try to recruit the best individual players, but rather recruit players who generated the most runs (even in indirect ways) and therefore wins.
The term is now used across sports for coaches and organizations using analytics to underpin new approaches to tactics and recruitment. Rather than old-fashioned “eye tests” or the use of rudimentary statistics like home runs, sports data science is incredibly complex and takes all sorts of metrics into account.
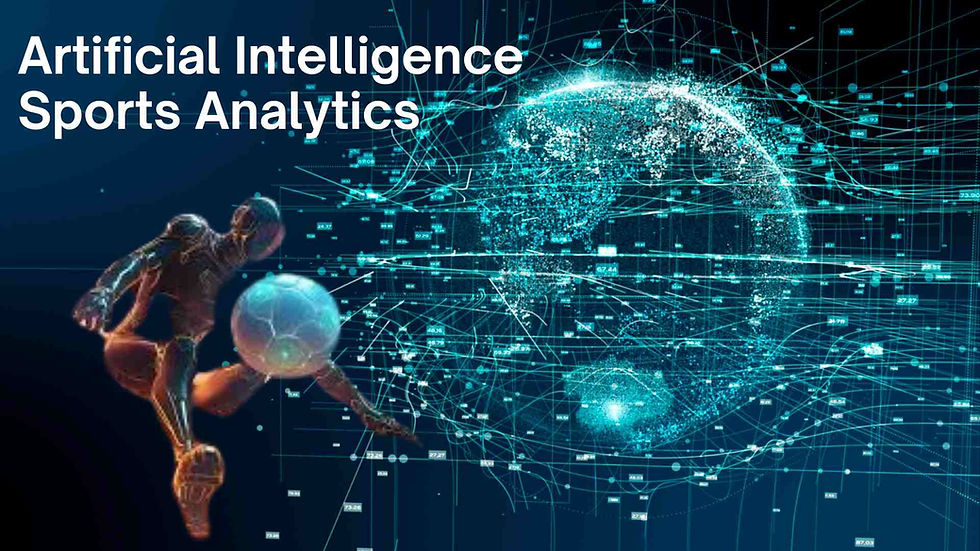
A very simple example is how you might measure the performance of a quarterback (QB) in American football. Basing your QB analysis solely on the number of team wins is clearly not insightful. It might capture some aspects of the player's contribution but at a minimum, the analysis will be clouded by the team’s defensive performance. The analysis could be refined by just looking at how many points the team scored and disregarding how many they conceded or allowed.
However, that still does not paint an accurate picture of the QB performance and you will see many in sports media discuss a QB based on the number of touchdowns and interceptions thrown (i.e. how many of his passes lead to a score and how many turned over possession to the opposition). This is clearly somewhat accurate. However, more advanced analysis of QB performance will look at metrics like catchable passes (passes that should have been caught, rather than ones that were actually caught) or EPA (Expected Points Added) and CPOE (Completion Percentage Over Expectation).
Advancing Sports Analytics With AI
As the analysis becomes more and more advanced, the scope for technology to play a role increases in parallel. The primary type of artificial intelligence used in sports analytics is machine learning and the analysis of “big data”. The ability of machines to find patterns is far beyond that of humans, particularly when it comes to analyzing vast amounts of empirical data. Similar to our stylized QB example above, AI-powered machine learning algorithms can help identify if a player scores a lot because he himself is exceptional or if he is reaping the reward of an exceptional player elsewhere on the team or the contribution of others.
Learn how computer vision and machine learning can be appied to pickleball AI performance analysis.
A good example in Association Football, or soccer, is the evolution of measuring a player’s attacking output. Originally a player would be judged on goals scored. Then the concept of assists was added, to measure not only scoring but facilitating other teammates scoring. However, this did not take into account poor finishing by other players so now teams will measure Expected Assists (xA). This is similar to the catchable pass in American football. If you pass the ball to a teammate and that pass should be scored 100% of the time (which, admittedly is unlikely) you would then receive an xA score of 1.0 - However if that pass should lead to a goal threequarters of the time it would give you an xA score of 0.75.
Types of Data in AI Sports Analytics
This type of analysis is often referred to as Event Data. You track the key events in a game, such as goals, passes and fouls in soccer, and then give the information to powerful machine learning software and it will identify patterns. Essentially this measures what happens in a game, when it happens, and what the patterns are.
However, we can take the analysis even further by adding another AI-powered technology, Computer Vision. This is the same software that underpins facial recognition technology. Essentially this technology converts camera images into analysable data. The application of computer vision in sport science is known as Tracking Data.
Sticking with our soccer analogy, let's consider what happens in the build-up to a goal. One player scores, one player gets an assist, and both of these will be measured by metrics such as xG (Excepted Goals) and xA (Expected Assists). However, what about players who contributed without touching the ball or generating an event (i.e. something that would be recorded as part of Event Data)? That is where Tracking Data comes in.
Soccer coaches are always training their players to make off-the-ball runs. This creates space for your teammates and can cause confusion among the opposition players. Through the use of cameras and computer vision AI can now track these runs and analyze their contribution to the team’s performance. While one player is scoring all the goals, others may be contributing significantly to this through selfless running or passing earlier in the build-up or pre-scoring phases.
This would be practically impossible without artificial intelligence. Even if we could see all 22 players on the field it is far beyond the capacity of a human brain to track and record so much simultaneous movement. With AI it is essentially automatic. Most professional sports games have many cameras and these are now using computer vision to convert the position and movement into Tracking Data and recording it for machine learning analysis.
The application is similar to the AI-powered Second Spectrum software used in the NBA. This artificial intelligence uses the same logic as discussed above, with computer vision software tracking the position of players and their actions and recording it to a huge database of games. Machine learning algorithms then analyze the data, giving coaches insights into how plays develop, how to defend better and so much more.
The use of AI in sports analytics is still in its infancy but it is already taking the in-game and performance analysis to the next level through computer vision and machine learning. What about other areas of sports science? Lets take a look at some other ways AI is being used to modernize sports analytics.
Artificial Intelligence and Injury Prevention in Sports
Injury prevention is a key component of sports science and as the value of players continues to increase teams are becoming more and more concerned about keeping them healthy. Using the same suite of technology as in performance analysis teams can predict injuries and make changes before a player is hurt. Obviously, not all injury is predictable but in some cases, there are telltale signs and AI can identify when a player is running an increased risk of injury.
One cutting-edge example is using computer vision to see when a player might begin to favor one side of his body over the other. For example, consider a player whose left ankle is weaker than his right. Computer vision will recognize when he starts to favor his right side before the human eye would, and that would be a warning sign that the risk of injury is too high. This is considered to be a leading indicator of an injury and as any sports fan, athlete or coach can tell you, prevention is better than cure.
Artificial Intelligence and Player Recruitment
Another area of sport being revolutionized by AI is player recruitment. Artificial intelligence helps coaches make better in-game decisions, better injury prevention decisions, and now we can see how it helps make better recruitment decisions. Using similar data and technology, artificial intelligence can help teams in two ways. Firstly, artificial intelligence can show teams what their true need is, and secondly, AI can reveal the true qualities a player has.
The Use of AI in The NFL Draft
Player recruitment is difficult in any sport but there is nothing quite as intense as the NFL draft. Pro Football in the US enjoys one of the most level playing fields in all of sport, thanks in no small part to the draft system. This sees the best 250 or so university players each year move to the NFL with the weaker teams getting the first choice of picks. Teams need to understand what their weaknesses are and have a plan of how to address those shortcomings by bringing in new talent. Artificial intelligence lets coaches see patterns in performance data, both of their own team and the players in the draft, and make better decisions.
Does a college quarterback throw the ball exceptionally well, or does he have receivers who are so good they make his mediocre passes look more impressive than they really are? That is a straightforward question and one that most American football coaches could probably answer themselves without the help of any technology. There are, however, many other similar questions where AI is far more advanced. The performance of fullbacks or running backs could require tracking the position and performance of many other players at the same time and is therefore more suited to computer vision.
Off-Field Decisions and On-Field Performance
Recruitment technology is now taking into account additional data beyond just game day performance. Just because a player is good it does not necessarily mean he will be good for you. Sport is littered with flops and stars who didn't cut it and that is often down to off-field factors as opposed to any physical or technical attributes. NFL teams are now incorporating player social media into their AI-powered recruitment algorithms to help better understand the players.
AI Recruitment in Soccer
Soccer teams spend billions of dollars every year buying players and many clubs seem to struggle to find the right fit. The Cristiano Ronaldo-inspired Saudi League is offering clubs a legitimate exit strategy for marquee signings that did not fit their system, but historically, overspending on a big-name player was a disaster for European football clubs. Unlike in American sports, in Europe players are paid whether they play or not, and it can be impossible to get an expensive player off your books.
English heavyweights Manchester United are currently suffering in this way having overpaid for the defender Harry Maguire. Maguire led Leicester to a history-making Premier League win in 2016 and he was considered one of the best English centre-backs. Then he made the move to England’s most successful team for a record £80 million pound fee and it looked a perfect move. Except it wasn’t. Almost nothing has gone right for Maguire at United and the club has tried to offload him multiple times but his wages are too high and he is almost unsellable.
Could AI have prevented this? Well, the truth is very probably, yes. Just because Maguire was a good defender in one system a Leicester it should not be assumed he would be as effective in Manchester, surrounded by different players, playing a different style, for a different coach.
This stands in stark contrast to the approach taken by far smaller clubs like Brentford. Inspired by their gambling and stats-obsessed owner Matthew Benham the London club have used Moneyball techniques to buy diamonds in the rough over and over again. This has allowed them to reach and stay in the English Premier League on a far smaller budget than their rivals. Their AI approach to recruitment would never allow them to waste £80 million on a big-name player who did not fit the system.
Artificial Intelligence in Sports Analytics
AI is changing every single industry and sport is no different. Computer vision allows us to record far more than the human eye can see, and machine learning identifies patterns hidden in the data. This is helping coaches and teams make better in-game decisions, prevent injuries, and recruit better. Some teams have leaned into this new frontier while others are slowed to adapt and the Rob Thomas quote comes to mind. The IBM Senior Vice President said, "AI is not going to replace managers, but managers who use AI will replace the managers who do not." Artificial Intelligence is not going to win football games, but football teams who use AI will win over teams who do not.
Want to learn more? Read how football teams use AI in areas such as recruitment and injury prevention.
Comentários